11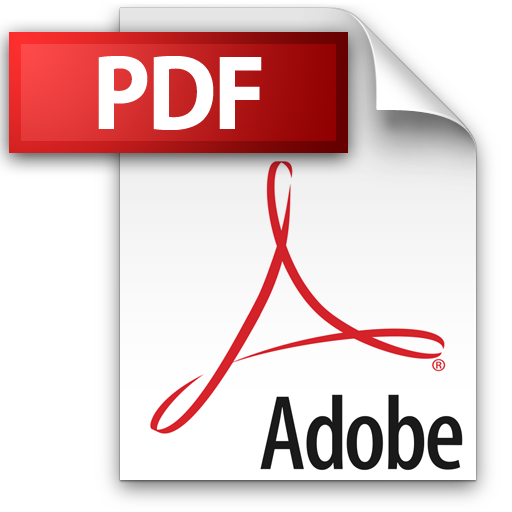 | Add to Reading ListSource URL: www.cis.pku.edu.cn- Date: 2016-10-19 02:44:00
|
---|
12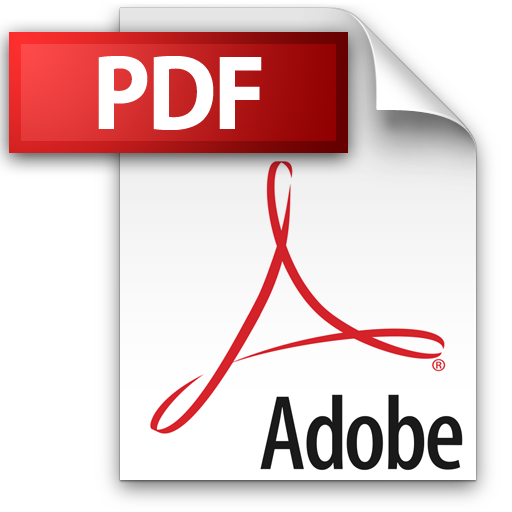 | Add to Reading ListSource URL: cse.iitk.ac.in- Date: 2016-09-03 04:33:30
|
---|
13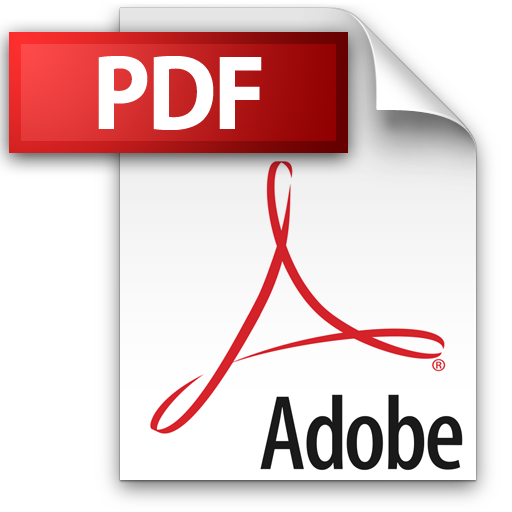 | Add to Reading ListSource URL: www.sci.unich.it- Date: 2013-12-10 01:36:05
|
---|
14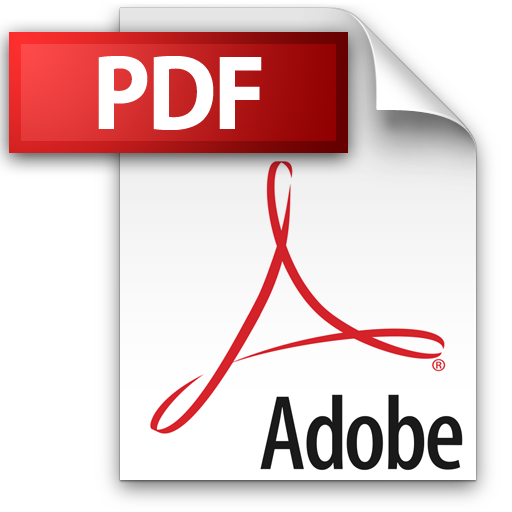 | Add to Reading ListSource URL: mmds-data.org- Date: 2016-06-23 15:50:48
|
---|
15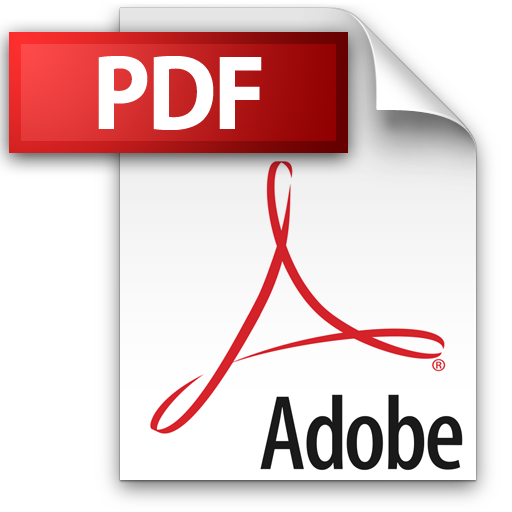 | Add to Reading ListSource URL: wp.nyu.eduLanguage: English - Date: 2016-08-16 16:20:31
|
---|
16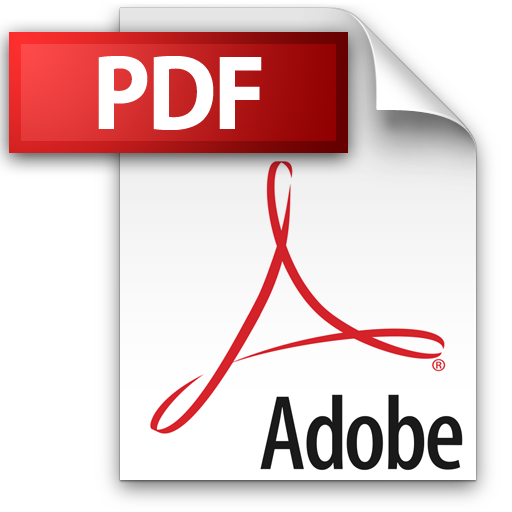 | Add to Reading ListSource URL: pmg.it.usyd.edu.auLanguage: English - Date: 2011-10-04 00:04:53
|
---|
17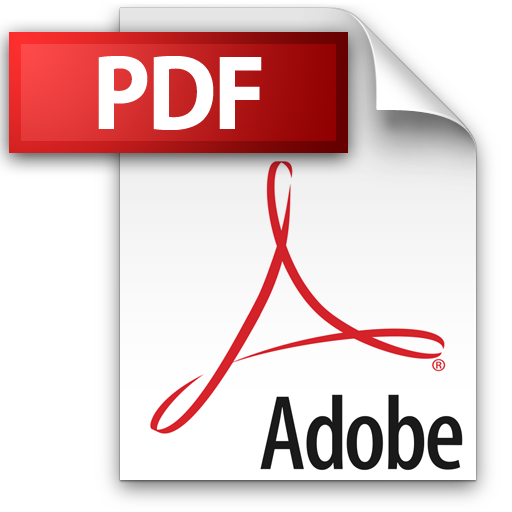 | Add to Reading ListSource URL: tedlab.mit.eduLanguage: English - Date: 2012-08-07 12:32:37
|
---|
18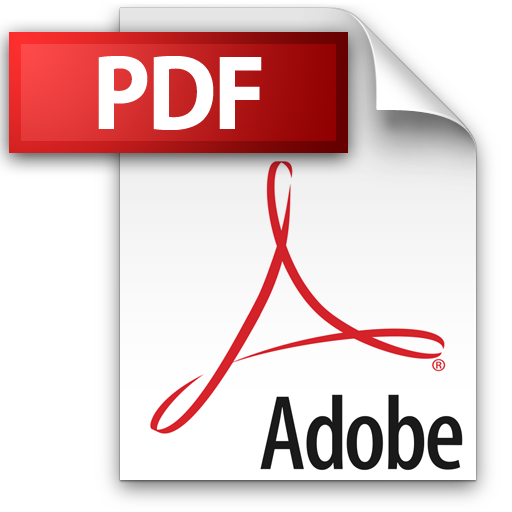 | Add to Reading ListSource URL: ulmo.ucmerced.eduLanguage: English - Date: 2009-09-24 14:04:55
|
---|
19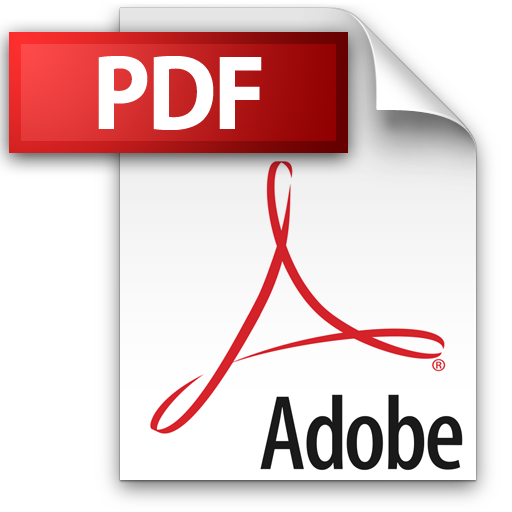 | Add to Reading ListSource URL: www.jsbi.orgLanguage: English - Date: 2002-12-09 05:53:39
|
---|
20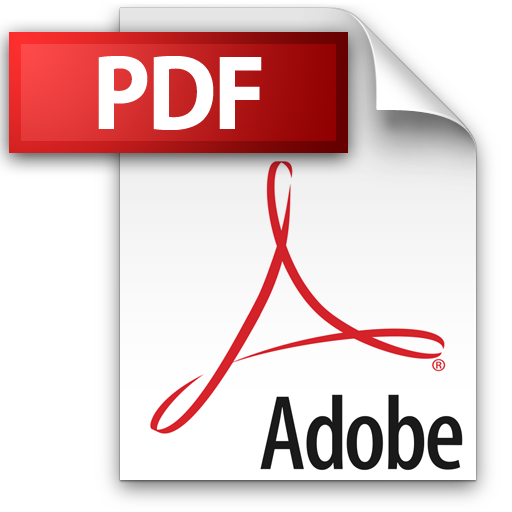 | Add to Reading ListSource URL: www.tepp.euLanguage: English - Date: 2015-07-01 09:59:09
|
---|